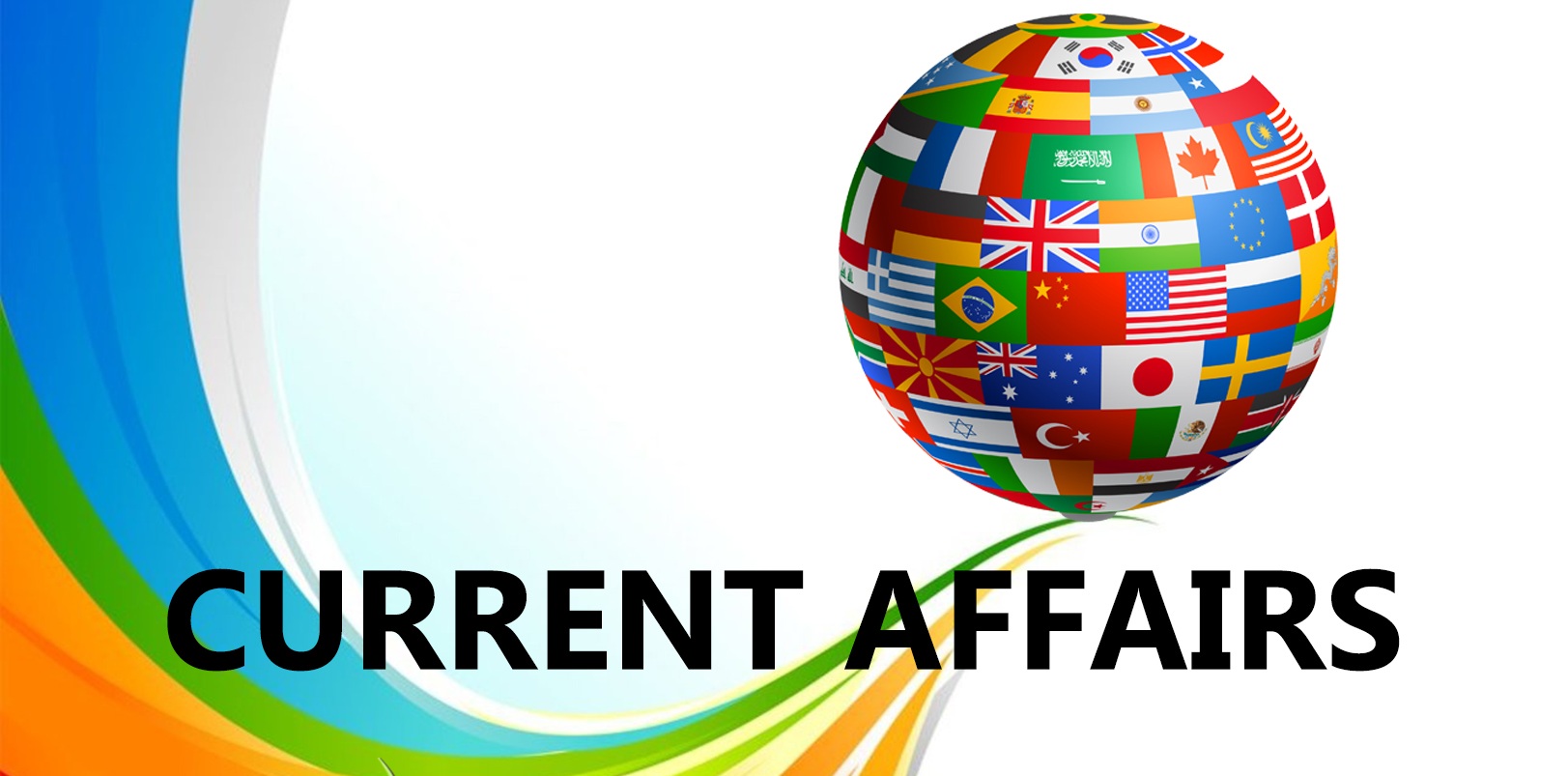
Multi-omics
About
Multi-omics is an integrative approach in biological research that involves the comprehensive study and combination of multiple types of "omics" data, such as genomics, transcriptomics, proteomics, metabolomics, and epigenomics. This approach provides a more holistic view of biological systems by examining the complex interactions between different molecular layers.
Key Components of Multi-Omics
- Genomics: The study of the entire genome of an organism, including its structure, function, evolution, and mapping.
- Transcriptomics: The study of the complete set of RNA transcripts produced by the genome under specific circumstances or in a specific cell.
- Proteomics: The study of the full set of proteins expressed by a genome, cell, tissue, or organism, including their structures and functions.
- Metabolomics: The study of the complete set of metabolites (small molecules) within a biological sample, providing insights into metabolic pathways and networks.
- Epigenomics: The study of the complete set of epigenetic modifications on the genetic material of a cell, which can affect gene expression without altering the DNA sequence.
Applications of Multi-Omics
- Disease Research: Helps in understanding complex diseases like cancer, diabetes, and cardiovascular diseases by identifying biomarkers and therapeutic targets.
- Personalized Medicine: Facilitates the development of tailored medical treatments based on individual genetic and molecular profiles.
- Drug Discovery: Assists in identifying new drug targets and understanding drug mechanisms.
- Agriculture: Improves crop breeding and resistance to diseases by understanding plant biology at multiple omics levels.
- Microbiome Studies: Investigates the complex interactions between host organisms and their microbial communities.
Challenges in Multi-Omics
- Data Integration: Combining data from different omics layers is computationally challenging and requires sophisticated bioinformatics tools.
- Standardization: Lack of standardized protocols and methods across different omics technologies.
- Data Volume and Complexity: Managing and analyzing large-scale omics data requires significant computational resources and expertise.
- Interpreting Results: Understanding the biological significance of multi-omics data and translating it into meaningful insights is complex.
Tools and Technologies
- Bioinformatics Software: Tools like Galaxy, Cytoscape, and others for data analysis and visualization.
- High-Throughput Sequencing: Technologies like next-generation sequencing (NGS) for generating large-scale genomics and transcriptomics data.
- Mass Spectrometry: Used in proteomics and metabolomics for identifying and quantifying proteins and metabolites.
- Machine Learning: Applied to integrate and interpret complex multi-omics datasets.
Multi-omics holds great potential for advancing our understanding of biology and improving healthcare outcomes through more personalized and precise approaches.